Sep
21
使用pprof和go-torch排查golang的性能问题
最近线上服务压力很大,api的p99有点扛不住。
广告业务对延时的要求普遍比较严格,有些adx设置的超时时间低至100ms,因此亟需找出性能热点。
根据对目前系统情况的估计(和metrics埋点数据),大致估计问题出在广告的正排环节。
使用 pprof 也证明了这一块确实是热点:
其中第三行 NewFilter 就是正排过滤函数。因为一些历史原因,系统里不是所有定向条件都使用了倒排,正排实现起来毕竟简单、容易理解,而一旦开了这个口子,就会有越来越多正排加进来,推测是这个原因导致了性能的逐渐衰退。
经过讨论,D同学花了一周多的时间,逐个梳理重写。在Libra(字节跳动内部的ABTest平台,参考谷歌分层实验框架方案)上开实验,平均耗时 -9%,从统计数据上来说,实验组比对照组有了显著的改进,但从最终结果上,整体的p95、p99超时都出现了进一步恶化。
这说明真正的问题不在于正排的计算,优化的思路出现了偏差。
考虑到晚高峰期间的cpu占用率也只是刚超过50%,也就是说有可能性能问题在于锁,但pprof的 block 和 mutex 都是空的,没有线索。
猜测问题有可能在日志,代码里确实用得不少。日志用的是 github.com/ngaut/logging 库,每一次调用都会用到两个全局mutex。但通过调整log level 为error级别,大幅减少了日志量,并没有看到性能的改善。
经过搜索,发现 uber 基于 pprof 开发了一个神器 go-torch,可以生成火焰图。安装好 go-torch 及依赖 FlameGraph 以后执行
用 Chrome 打开 cpu.svg,人肉排查:
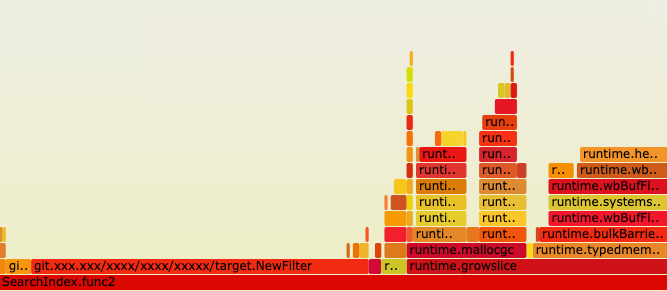
可以看到,在NewFilter旁边竟然还有一个耗时接近的 runtime.growslice ,结合实际代码(略作简化),可以推测是 slice 的初始化长度不足。
实际上最终定向后得到的广告往往在数万甚至数十万的级别,而 go 的 slice 扩容在超过1024个元素以后是1.25倍,可想而知会出现大量的内存分配和拷贝,导致性能随着广告数量的增加逐渐恶化。最近的广告数量也确实有了大幅的上升 —— 逻辑上形成了闭环。
经过优化,使用更大的初始化长度,并且使用 sync.Pool 来进一步减少内存分配,最终上线后p95和p99都下降了超过50%,效果显著。
参考:
golang 使用pprof和go-torch做性能分析 https://www.cnblogs.com/li-peng/p/9391543.html
转载请注明出自 ,如是转载文则注明原出处,谢谢:)
RSS订阅地址: https://www.felix021.com/blog/feed.php 。
广告业务对延时的要求普遍比较严格,有些adx设置的超时时间低至100ms,因此亟需找出性能热点。
根据对目前系统情况的估计(和metrics埋点数据),大致估计问题出在广告的正排环节。
使用 pprof 也证明了这一块确实是热点:
引用
$ go tool pprof http://$IP:$PORT/debug/pprof/profile
...
(pprof) top 10
Showing nodes accounting for 25.50s, 32.63% of 78.14s total
Dropped 1533 nodes (cum <= 0.39s)
Showing top 10 nodes out of 284
flat flat% sum% cum cum%
4.56s 5.84% 5.84% 4.87s 6.23% syscall.Syscall
4.03s 5.16% 10.99% 4.03s 5.16% runtime.aeshashbody
3.50s 4.48% 15.47% 6.01s 7.69% git.xxx.org/xxx/target.NewFilter
2.78s 3.56% 19.03% 3.73s 4.77% runtime.mapaccess2_fast64
2.63s 3.37% 22.40% 4.52s 5.78% runtime.mapiternext
2.08s 2.66% 25.06% 2.16s 2.76% runtime.heapBitsForObject
1.65s 2.11% 27.17% 1.93s 2.47% runtime.mapaccess1_fast64
1.57s 2.01% 29.18% 2.96s 3.79% runtime.mapaccess2
1.43s 1.83% 31.01% 2.06s 2.64% runtime.runqgrab
1.27s 1.63% 32.63% 1.27s 1.63% runtime.epollwait
(pprof) png
Generating report in profile001.png (使用生成的线框图查看耗时)
...
(pprof) top 10
Showing nodes accounting for 25.50s, 32.63% of 78.14s total
Dropped 1533 nodes (cum <= 0.39s)
Showing top 10 nodes out of 284
flat flat% sum% cum cum%
4.56s 5.84% 5.84% 4.87s 6.23% syscall.Syscall
4.03s 5.16% 10.99% 4.03s 5.16% runtime.aeshashbody
3.50s 4.48% 15.47% 6.01s 7.69% git.xxx.org/xxx/target.NewFilter
2.78s 3.56% 19.03% 3.73s 4.77% runtime.mapaccess2_fast64
2.63s 3.37% 22.40% 4.52s 5.78% runtime.mapiternext
2.08s 2.66% 25.06% 2.16s 2.76% runtime.heapBitsForObject
1.65s 2.11% 27.17% 1.93s 2.47% runtime.mapaccess1_fast64
1.57s 2.01% 29.18% 2.96s 3.79% runtime.mapaccess2
1.43s 1.83% 31.01% 2.06s 2.64% runtime.runqgrab
1.27s 1.63% 32.63% 1.27s 1.63% runtime.epollwait
(pprof) png
Generating report in profile001.png (使用生成的线框图查看耗时)
其中第三行 NewFilter 就是正排过滤函数。因为一些历史原因,系统里不是所有定向条件都使用了倒排,正排实现起来毕竟简单、容易理解,而一旦开了这个口子,就会有越来越多正排加进来,推测是这个原因导致了性能的逐渐衰退。
经过讨论,D同学花了一周多的时间,逐个梳理重写。在Libra(字节跳动内部的ABTest平台,参考谷歌分层实验框架方案)上开实验,平均耗时 -9%,从统计数据上来说,实验组比对照组有了显著的改进,但从最终结果上,整体的p95、p99超时都出现了进一步恶化。
这说明真正的问题不在于正排的计算,优化的思路出现了偏差。
考虑到晚高峰期间的cpu占用率也只是刚超过50%,也就是说有可能性能问题在于锁,但pprof的 block 和 mutex 都是空的,没有线索。
猜测问题有可能在日志,代码里确实用得不少。日志用的是 github.com/ngaut/logging 库,每一次调用都会用到两个全局mutex。但通过调整log level 为error级别,大幅减少了日志量,并没有看到性能的改善。
经过搜索,发现 uber 基于 pprof 开发了一个神器 go-torch,可以生成火焰图。安装好 go-torch 及依赖 FlameGraph 以后执行
引用
$ go-torch -u http://$IP:$PORT -f cpu.svg
INFO[14:52:23] Run pprof command: go tool pprof -raw -seconds 30 http://$IP:$PORT/debug/pprof/profile
INFO[14:52:54] Writing svg to cpu.svg
INFO[14:52:23] Run pprof command: go tool pprof -raw -seconds 30 http://$IP:$PORT/debug/pprof/profile
INFO[14:52:54] Writing svg to cpu.svg
用 Chrome 打开 cpu.svg,人肉排查:
可以看到,在NewFilter旁边竟然还有一个耗时接近的 runtime.growslice ,结合实际代码(略作简化),可以推测是 slice 的初始化长度不足。
matchAds := make([]*ad, 0, 4096)
adsBitMap.GetList(func(seq int) {
if NewFilter(ctx, ad) {
matchAds = append(matchAds, adlist[seq])
}
})
// 顺便提一下,bitmap是一个uint64数组,GetList(f) 是将每一个等于1的bit索引传给 f
// GetList方法里面用了cpu的BSF指令来提高性能。
adsBitMap.GetList(func(seq int) {
if NewFilter(ctx, ad) {
matchAds = append(matchAds, adlist[seq])
}
})
// 顺便提一下,bitmap是一个uint64数组,GetList(f) 是将每一个等于1的bit索引传给 f
// GetList方法里面用了cpu的BSF指令来提高性能。
实际上最终定向后得到的广告往往在数万甚至数十万的级别,而 go 的 slice 扩容在超过1024个元素以后是1.25倍,可想而知会出现大量的内存分配和拷贝,导致性能随着广告数量的增加逐渐恶化。最近的广告数量也确实有了大幅的上升 —— 逻辑上形成了闭环。
经过优化,使用更大的初始化长度,并且使用 sync.Pool 来进一步减少内存分配,最终上线后p95和p99都下降了超过50%,效果显著。
参考:
golang 使用pprof和go-torch做性能分析 https://www.cnblogs.com/li-peng/p/9391543.html
欢迎扫码关注:
转载请注明出自 ,如是转载文则注明原出处,谢谢:)
RSS订阅地址: https://www.felix021.com/blog/feed.php 。
miuc

2019-11-4 11:12
最近也在用golang写番羽算法,但近期懒癌发作。。看来是要逼着赶紧写完了。。
分页: 1/1
1

